Saving $80K+ with employee questionnaire automation
We built a NLP-based neural network designed to automate question generation, saving $80K+ in the first year for one of Canada’s leading HR software businesses.
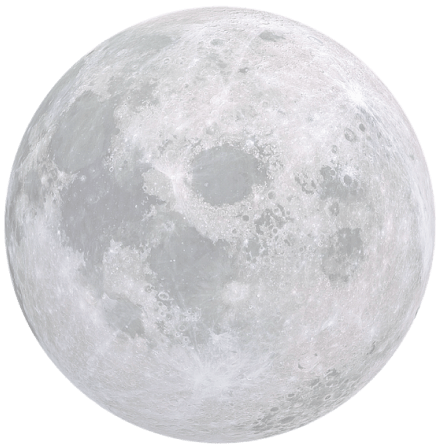
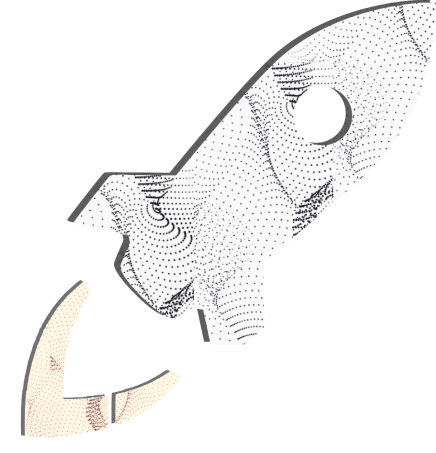
The Client
A SaaS company providing solutions for digital onboarding, employee records, time-off management, automated benefits, and payroll synchronization. Among other things, the software enables businesses to onboard new employees, complete tax forms, sign and store employment documents electronically, set up and track time-off policies, automate and manage payroll.
The Challenge
One of the key promises of the client’s solution is its ability to understand and account for personal circumstances of each employee, ensuring ease of use and employees’ satisfaction.
While employees use benefits plans in a somewhat similar way, each employee’s use case often involves a circumstance that needs to be accounted for to enable the desired degree of personalization. Dealing with multiple third-party insurers and benefit providers, the client employed several people whose job was to read benefits brochures, insurance policies and similar content, identify provisions relevant to specific use cases and update the knowledge base with each new scenario. Given that policies frequently change, maintaining the knowledge base represented a significant cost for the client.
The Solution
Crater Labs proposed an NLP-based neural network capable of composite understanding of the text in order to formulate context-aware questions and provide intelligent responses.
The research project benefited the client in multiple ways. In addition to greatly reducing human involvement in the development and maintenance of the knowledge base, it enabled the client to develop a self-learning question/answer chatbot, extract relevant benefits data and automatically generate/update benefit FAQ, and enable intelligent content analysis to identify cross-selling opportunities.
IP Generated
Crater Labs developed an RNN encoder-decoder architecture with the global attention mechanism enabling the model focus on certain elements of the input when generating each word during decoding and enabling intelligent context extraction. The model outperforms commercially available solutions.
Benefits & ROI
This project resulted in ~$80,000 annual cost savings in addition to more than 100% return on investment from leveraging Canadian investment tax credits. This ROI does not account for the potential value of the developed IP.
Subscribe
Sign up with your email address to receive news and updates
More Moonshots Worth Celebrating
Minimizing radiation exposure in construction projects
We developed a custom LSTM neural network to predict radiation exposure of construction workers in sensitive sites, improving shift scheduling and safety compliance.
Read More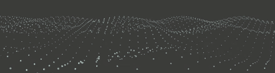
Eliminating foreign accent bias with video recorded interviews
We used deep learning to improve job applicant videos for an online recruiter, deciphering foreign accents and eliminating bias in the process.
Read More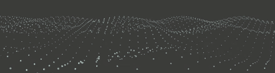
Saving $80K+ with employee FAQ generation
We built an NLP-based neural network designed to automate question generation, saving $80K+ in the first year for one of Canada’s leading HR software businesses.
Read More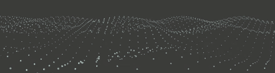