Energy demand prediction for generation management
We developed a deep reinforcement learning model to assist energy producers in scheduling maintenance and minimizing operating costs.
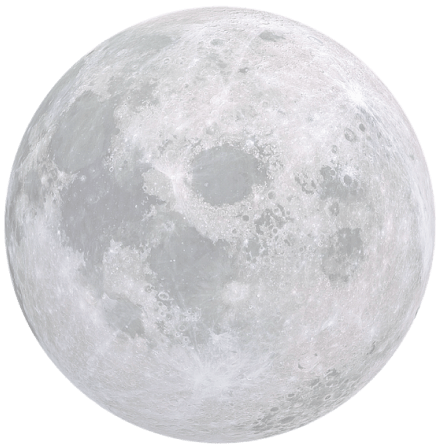
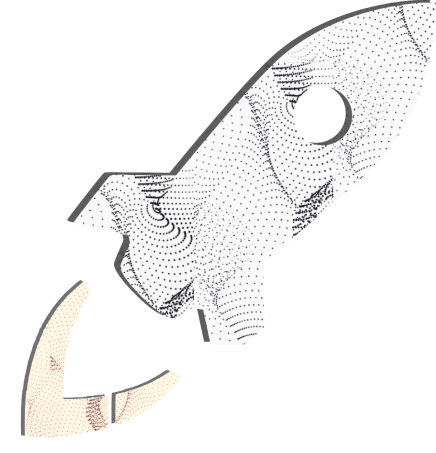
The Client
A multinational company involved in producing, selling and delivering electricity and related products and services in various markets to a diverse group of customers, representing commercial, government and retail sectors. The client operates several power-generating facilities.
The Challenge
Power plant maintenance often results in a reduction of the plant’s output. As such, it requires time-consuming coordination and can be costly if not properly planned. To minimize the costs associated with plant maintenance, our client sought to develop a model that could help identify windows of opportunities to conduct maintenance when power demand or power prices were lower.
The Solution
Our client had implemented a model to predict daily and weekly power output requirements using standard machine learning models. This model had a ~75% accuracy (with confidence scores over 90%) in predicting the power output level required for the given time windows. We sought to improve upon this model by employing a recurrent neural network (RNN) and adding auxiliary data to their model, such as commercial billing data, data on humidity and air quality, along with related utility consumption data to generate more accurate insights.
IP Generated
Crater Labs developed a recurrent neural network that uses a custom-designed reward function that accounts for downtime cost, allowing our client to process larger volumes of data and generate more nuanced results. This model, along with the visualization dashboard we created, gives our client a tool to minimize the costs associated with maintenance downtime. Our model improved prediction accuracy to over 85%, and we expect the prediction accuracy of the model to improve further, as it is fed more data.
Benefits & ROI
Our client has reduced lost revenue due to power plant maintenance by hundreds of thousands of dollars. The model will be used across a number of power plant maintenance projects over the coming months and years and is projected to provide savings in the millions of dollars.
Subscribe
Sign up with your email address to receive news and updates
More Moonshots Worth Celebrating
Minimizing radiation exposure in construction projects
We developed a custom LSTM neural network to predict radiation exposure of construction workers in sensitive sites, improving shift scheduling and safety compliance.
Read More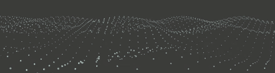
Optimizing product placements in print flyers to maximize revenue
We combined a convolutional neural network with a custom region proposal network that provides key insights on how to maximize revenue with flyer layout, with a double-digit return expected within the first two years.
Read More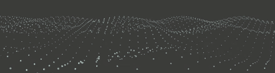
Identifying Potential Security Access Violations
We developed a semi-supervised neural network to identify anomalous security access card swipes in a large corporation with numerous facilities throughout the world, previously hidden secure access violations.
Read More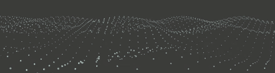